Opinionated SEO Opinions™
Tackling myths, misconceptions, and common FAQs about Search Engine Optimization. Beware: opinions ahead!
Listen & Subscribe
Watch our latest episode
Want to ask an SEO question?
Reach out today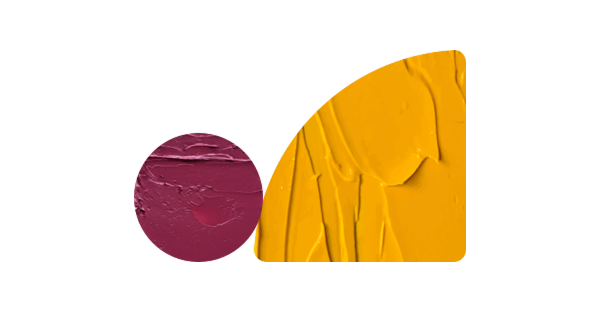
Tackling myths, misconceptions, and common FAQs about Search Engine Optimization. Beware: opinions ahead!